„Our experience has taught us that if your organization hasn’t created and thoroughly tested, repeatedly, a cyber incident response plan across all business areas and personnel, as well as performed simulations of cyber attacks, you won’t do a good job of responding when it occurs for real. We see over and over that it is very difficult to make good decisions when you’re responding to a real attack in the heat of the moment.” /David Burg, Cyber Security & Privacy Leader PwC/
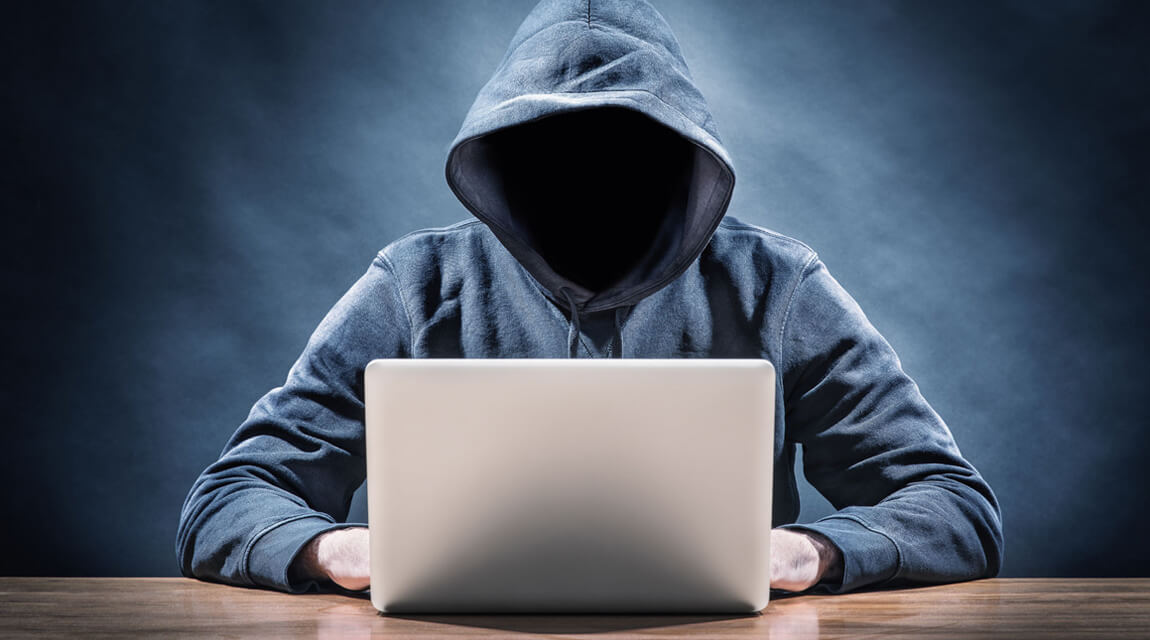
10 (MORE THAN) ALARMING FIGURES!
- At present, more than 50% of jobs involve using a computer which is certain to reach 77% by the year 2020, Global Economic Crime Survey 2016 (PwC): 36% of organizations have already experienced various form of economic fraud, cybercrime being the second most commonly reported on the list,
- 34% of businesses expect to be affected by it in the foreseeable future,
- In 2016 alone, 54% of economic crime in the US was connected with cybercrime, comparing to 44% in 2014,
- In case of each of 9% of British firms affected by cybercrime, their loss exceeded $1m, with 20% losing between $100 000 and $1m,
- 56% of Indian companies realise the increased risk of cybercrime over the past two years,
- The rate of fraud in case of Internet transactions is 12 times higher than it is within traditional in-store trade,
- Another finding is that 1 in 10 of fraud is discovered accidentally. So, how many are never to be discovered?
- 1 in 10 of American respondents have never conducted a fraud risk assessment, while 20% have done it only once in the last two years,
- 61% of CEOs are seriously worried about their companies’ cyber security yet at the same time only 37% of institutions already have a cyber incident response plan.
WE PUSHED THE BOULDER DOWN THE HILL
There could be no doubt: we witness the most logical though, at the same time devastating kind of relationship. Together with the world unstoppably moving into the cyberspace, so does this of crime, moreover, doing it with unprecedented velocity. In the US, for example, , it already takes the second place on the list of the most commonly reported, with the prospect of becoming the leader in the foreseeable future. Here is one more figure to display the scale of the problem: in 2012 alone (according to Polish daily “Rzeczpospolita”), over 19 000 crimes in the cyberspace were notified, while by only the first half 2016, the figure has grown to 25 824, out of which 17 196 (66%) were actually proven! Interestingly enough, the figures with reference to economic crimes account to 84.4%, drug cases to 96% and road accidents – 98.7% respectively. Far from comforting indeed!
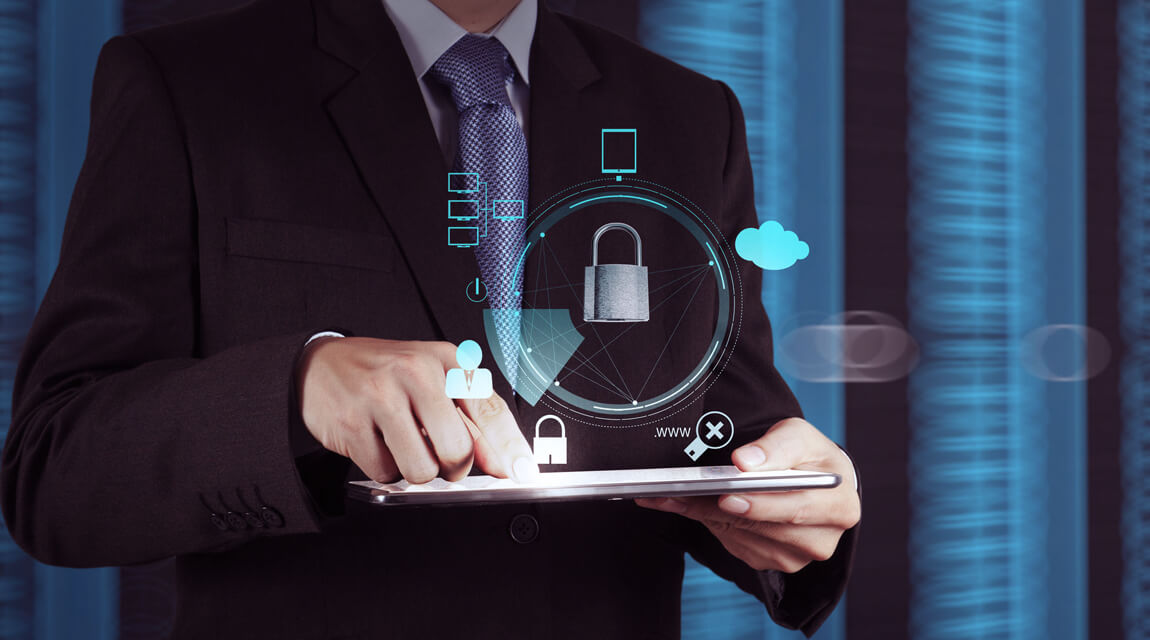
WE ARE NOT DEFENCELESS
The evolution of the wide range of cyber crime has been going step in step with the development IT technology. Its attacks embrace a number of fields such as telephone companies, insurance companies, tax returns, credit card transactions, as well as the retail industry both in traditional „in-shop” sense and, even more severely, the Internet transactions. Yet, first of all, there is banking industry which occurs to be the most vulnerable to crime and the possible losses the highest. A contemporary criminal is no longer a robber, wearing a black balaclava, equipped with a ladder, a crowbar and possibly a rifle…. He has been replaced by an invisible, highly-specialized IT specialist, who does his „job” quickly, and then disappears in no time!
It comes as little surprise then that the effective defence and protection against the cybercrime requires complex, long-lasting and tedious investigations which harness not only IT technology but other, seemingly distant domains of knowledge such as financial economies, business practices and law.
One of the pioneers in the discussed field was FICO Falcon (the company based in San Jose, California, with numerous branches all over the world) which implemented machine learning based on neural network shell. TJ Horan, vice president for fraud solutions at FICO said: „Consumer convenience is driving rapid growth in online transactions. As a result, criminals are looking to use this convenience to their advantage as chip cards and other security features have made physical fraud more difficult. Our goal is to help card issuers promote a positive consumer experience while protecting them from financial harm. These CNP machine learning innovations are important tools to help issuers spot fraud faster, and take on even greater importance in the light of recent data breaches, which will lead to more fraud attempts.” Actually, FICO proved to be highly successful since with the analysis of 4 billion transactions, they managed to cut CNP fraud losses by 30% as well as doubled the detection of fraudulent transactions on the first attempt!
Prevention and detection of fraud includes Knowledge Discovery in Databases, Data Mining, Machine Learning and Statistics while among the basic techniques applied statistical techniques and artificial intelligence should be mentioned.
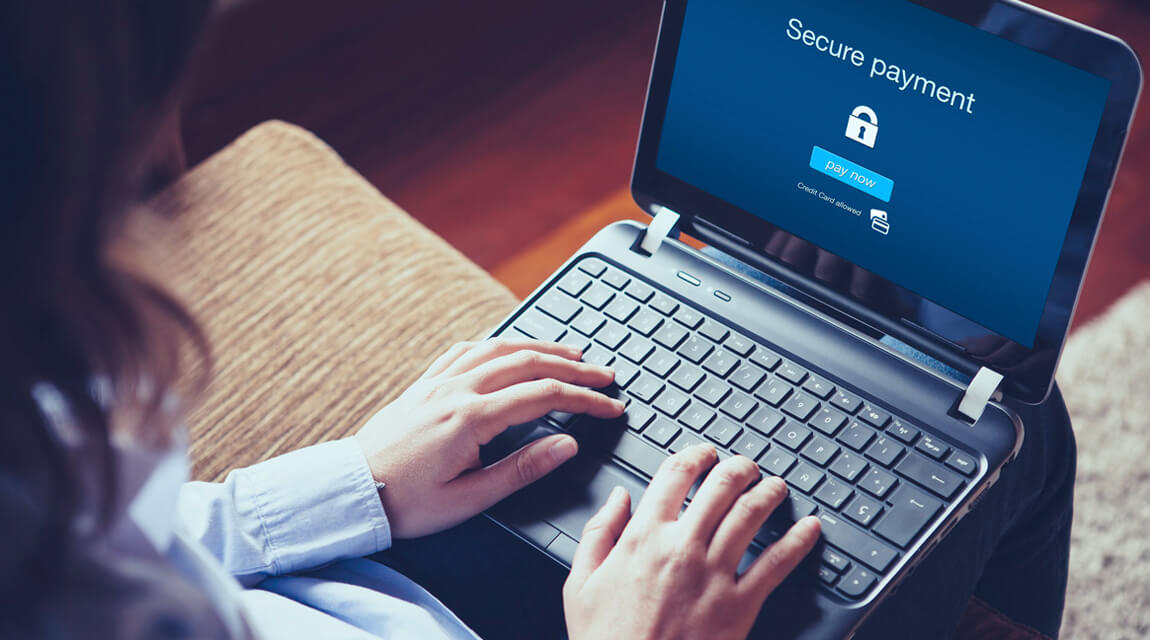
ABC OF MACHINE LEARNING
To put it in a nutshell, Machine learning, as a branch of computer science, provides the computer with the ability to learn with no need to be explicitly programmed. The term was coined over a half century ago (1959) by Arthur Samuel who contributed to the advent of computer gaming and Artificial Intelligence which explore and construct the algorithms that are able to draw knowledge and make predictions on the basis of the data. Machine learning can be applied in the wide range of tasks including e-mail filtering, detection of network intruders, attempts working towards data breach or optical character recognition. There is a close relationship between Machine Learning and Computational Statistics. This way Machine Learning enters the field of fraud detection since by means of overlapping its activity with Computational Statistics it can not only learn but also establish behavioural profiles for a range of units which can be subsequently used to detect anomalies which, in turn might give rise to fraud suspicion. It could be, for instance the review of employee’s credit card activity in order to assess the possibility of it being used for personal purposes. At this point it should be categorically stated that such sort of anomalies creates by no means a legally supported proof that a crime has been actually committed however, it may give rise to a suspicion which subsequently requires further examination. Therefore, in order to go beyond a method of dealing with data analysis, the system must be supplied with the background knowledge and be capable of effectively performing reasoning assignments taking advantage of the that data. The Machine Learning task can be referred to as processing the background knowledge as well as examples (input) into the real knowledge (output), which was initially hidden in the huge amount of data, and as such hardly detectable.
The findings gathered by Machine Learning and Artificial Intelligence can be divided into the supervised and unsupervised ones, both of which searching for entities such as accounts, customers, suppliers and the like, that show „unusual behaviour” and such anomalies create a suspicion field, which, once again, is only an indication of likelihood that the act of fraud has actually occurred. Thus, a sudden appearance of $2m on somebody’s account, against all the odds, does not automatically mean that the fraud must have been committed as the money might have been as well inherited or won on the lottery!
As far as the unsupervised model is concerned, it comprises a random sub-sample of records which is then manually classified as potentially fraudulent or non-fraudulent and then applied to train a supervised Machine Learning algorithms, which as a result lead to detect the records which prove to be fraudulent. Regarding the unsupervised machine learning methods, they do not deal with marked records. Instead, they detect individual entities that give signs of anomalous behaviour which is different from that previously assessed as similar.
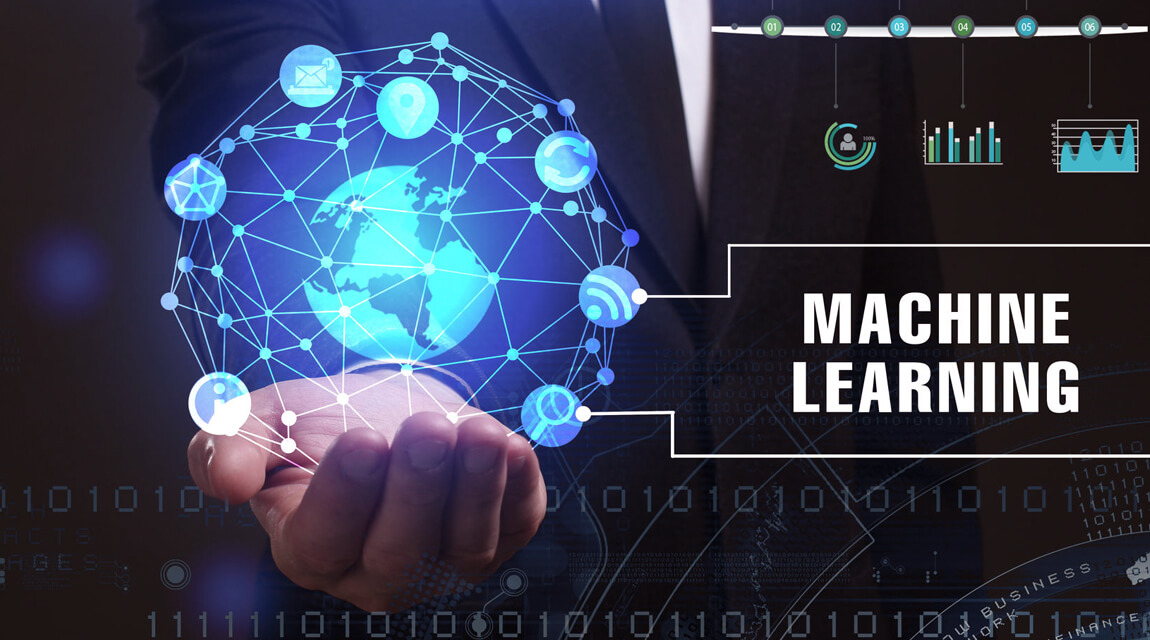
THE NATURAL ORDER
In practice the sequence of actions runs in the following way:
- Unsupervised models pick up a cluster of anomalies. Their role is to identify the average distribution of data e.g. the typical settings for iPhone user. This facilitates the establishment of baselines which after being grouped into clusters enables training the model in recognizing transactions as “good” or “bad” (potentially fraudulent). The knowledge of the baselines makes it possible to spot any anomalies which will require the further investigation.
- The findings are manually reviewed and labelled. At this phase the “suspicious”/anomalous transactions are manually examined in order to determine whether they are fraudulent or non-fraudulent
- The labels are fed into a supervised model in order to train it towards it being able to recognise bad transactions on its own. Needless to say, training models involve using huge amount of data which, in turn means that they are useful and effectively practical in case of large companies or those that process plenty of data points.
Gradually, owing to the training process as well as the growing amount of data, the model, like it is in case of a human student, gains experience and its efficiency is constantly growing. Thus, manual review becomes unnecessary and transactions are shifted directly from an unsupervised model to a supervised one.
Simility.com provides the following example of the whole flow: The case referred to a certain client which was a hotel listing app. The unsupervised model correctly identified some customers having their phones set in flight mode but then turning on Wi-fi trying to rent rooms. Yet, after the data being examined by human analysts, it turned out that they were on their business trips to foreign countries and booked the hotel rooms from the airports. The most likely reason for applying flight mode was to avoid the high cost of roaming. Subsequently, the supervised models were trained to allow such transactions as entirely non-fraudulent despite being anomalous at the same time.
The quoted case reveals one more significant aspect of any Machine Learning process which aims at fraud detection and this is the fact that apart from identifying harmful activity, they must also allow the proper operations to go through smoothly, with the view of the whole system providing exclusively positive impression on the users.
CONCLUSION: ARE WE STILL NEEDED?
Everything said above might suggest that fraud detection can be conducted in a fully automated way with no human participation. Such thinking is certainly false as, after all, it is human intelligence that unstoppably invents new, more and more sophisticated method of fraud. Therefore, the process of detection still requires human attendance on the other end of the stick. This is mainly because the instances of these new fraudulent actions must be fed into machines as fast as possible so the training process is to be constantly updated. Interestingly enough, still over 90% platforms dealing with fraud detection, refer to human reviewers, showing the strong attachment to traditional methods, despite the fact that training the specialists is time-consuming and expensive. However, the future belongs to machines, taking into account the growing number of transactions which the humans will not be able to process! Machines, despite their limitations are better than humans at reviewing huge amount of data. The three most important factors in this respect are:
- Speed – business demands immediate results, it is more often than once the matter of seconds! This is only feasible with the application of machines which work with incomparable to human velocity.
- Scale – together with the growth of databases, machines are not only more effective but their activities also embrace a vast scale of cases of fraud. Moreover, with time they become capable of predicting the possible movements of fraudsters in the future.
- Efficiency – unlike humans, machine can perform repetitive tasks. They are also more effective in dealing with and detecting subtle or non-intuitive patterns of fraudulent behaviour. Last but not least, unsupervised models can continuously analyse and process new data, thus becoming subjected to the constant updating process.
Let it be one more quotation to conclude, providing some food for thoughts: „Whilst the SFO is not in the business of giving advice, the best ethic and anti-corruption programmes are surely those which are simply stated, inculcation by training, energically enforced and lived by those in authority. A thick policy book, carefully lawyered but ignored in practice is as bad as no policy at all.” (David Green, Director, Serious Fraud Office)